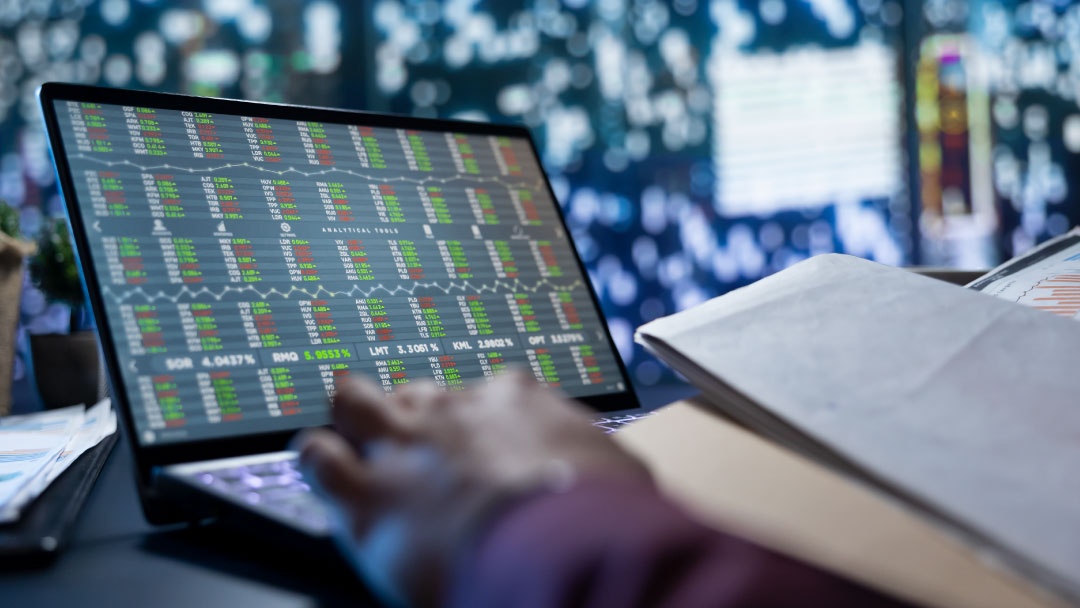
The application of growth models in aquaculture enables the prediction of species development, feed optimisation, and better resource management throughout the production cycle, thereby enhancing the sustainability of the industry. One widely used model is the von Bertalanffy model, initially developed for livestock in 1934 and now broadly applied in aquaculture.
In contrast to methods like the Logistic or Gompertz Models, the von Bertalanffy approach is distinctive for its focus on anabolic and catabolic processes, providing a more precise description of individual growth over time, especially for species with asymptotic growth patterns.
With advancements in AI, the integration of machine learning with traditional growth models overcomes many of their previous limitations. By incorporating real-time data and dynamically adjusting growth predictions, these systems improve both accuracy and adaptability to changing conditions.
AI Optimises Growth Models
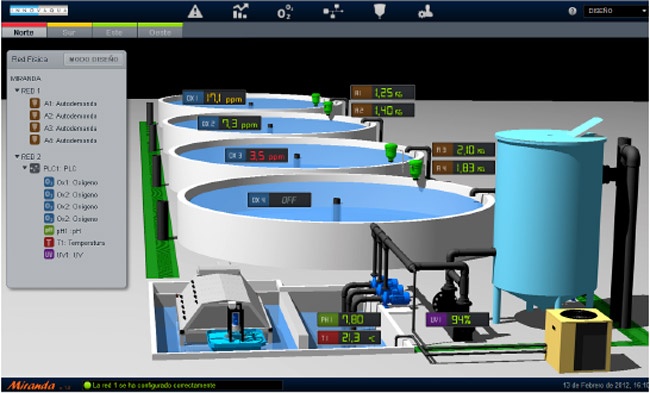
AI has the capability to process vast amounts of data, identifying growth patterns and adjusting for variables such as temperature, water quality, feed, and stocking density. When combined with a growth model like von Bertalanffy, AI enables highly accurate growth predictions, which is especially valuable in aquaculture environments, where conditions can change rapidly, impacting species growth.
Feed is one of the main costs in aquaculture, and AI can optimise it significantly. Through machine learning algorithms, AI systems can automatically adjust feed amounts based on fish size, growth rates, and water conditions. This not only enhances growth but also reduces feed waste and, consequently, environmental impact.
AI can integrate with Internet of Things (IoT) technology, such as sensors and cameras, enabling real-time monitoring of environmental conditions and fish health. This allows aquaculturists to make immediate adjustments to feeding or management practices, increasing efficiency and reducing losses. Continuous monitoring also aids in detecting health or water quality issues before they become major threats to production.
Costs and Challenges of Implementing AI and Growth Models
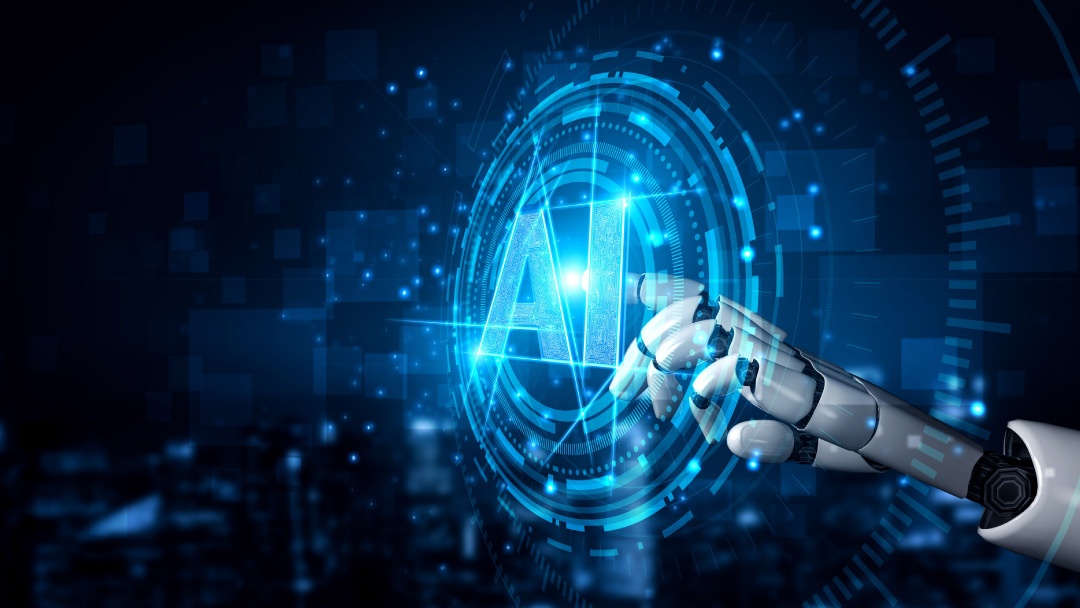