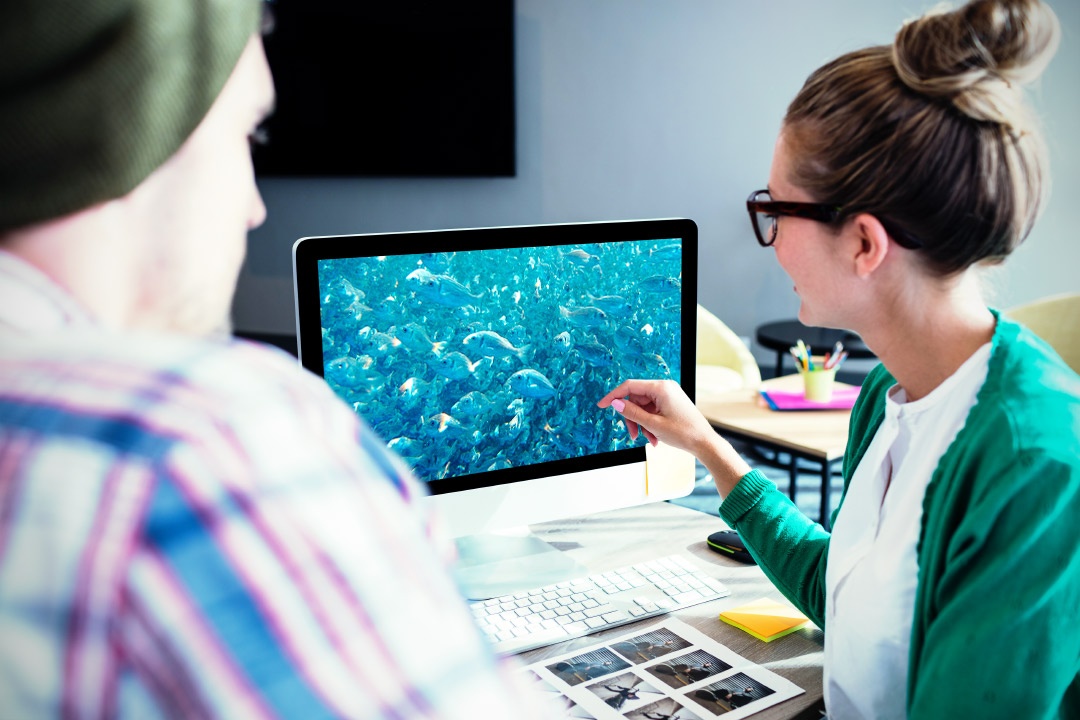
Artificial intelligence, when applied to aquaculture, opens up a wealth of opportunities, particularly though object detection models in computer vision. These models are designed to automatically identify and locate objects within images or videos, making them ideal for monitoring fish behavior in both closed recirculating aquaculture systems (RAS) and open marine net pens.
Among the most promising applications, are those focused on fish welfare, health, and feeding patterns.
Unlike human abilities, these models can continuously process vast amounts of data in real-time, identify subtle behavioural patterns or physical changes in first that might escape the human eye, and operate round the clock without the limitations of fatigue or subjectivity.
Popular models in the field include YOLO (Yoy Only Look Once), Faster R-CNN, and SSD. These are used to monitor fish health, behavior patterns, and disease prevention.
Depending on the technique used, models are divided into one-stage approaches like YOLO, SSD, and RetinaNet, which are excellent for real-time detection-such as ongoing fish monitoring in marine cages or RAS. On the other hand, two-stage models like Faster R-CNN, Mask R-CNN, and Detectron2 provide greater accuracy and are better suited for complex tasks or scenarios with overlapping objects, such as detailed fish analyses in labs or densely populated images with multiple individuals.
How Do You Choose the Right Model for Your Project?
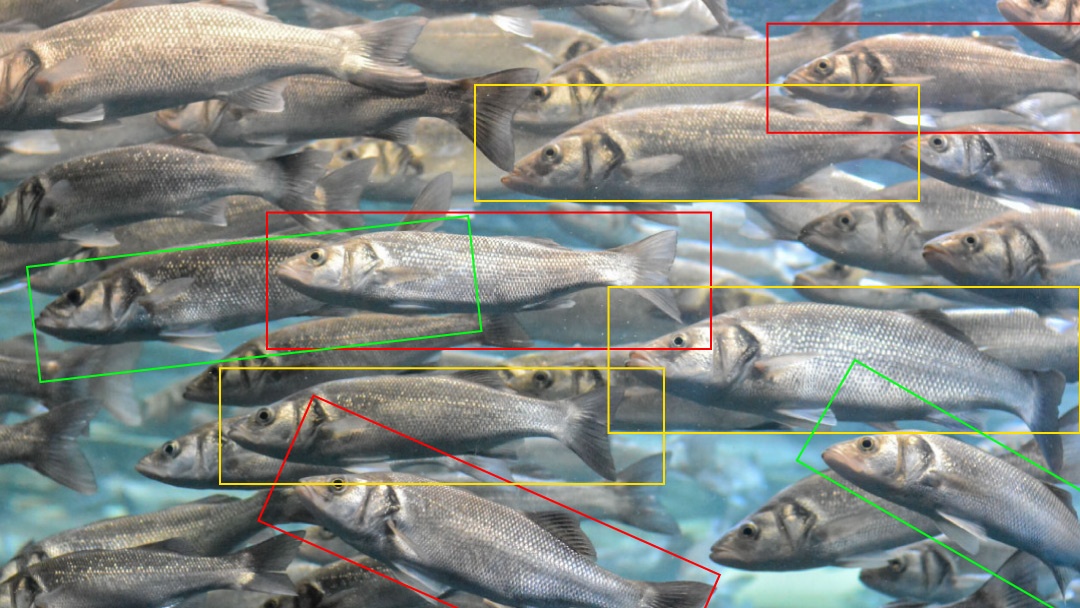
Choosing the correct model can significantly impact the success of aquaculture operations. The decision depends on the specific needs and technical constraints of the environment where it will be implemented. Striking the right balance between speed, accuracy, and available resources is essential.
For example, in marine floating cages, where continuous monitoring is critical, YOLOv5 has been employed to detect and count fish in real-time. This enables operators to adjust feed quantities based on fish density, optimizing resources and cutting costs. Similarly, YOLOv4 has been used in RAS to identify abnormal fish behavior, such as erratic movements, which could signal stress or disease.
When precision is paramount, two-stage models like Faster R-CNN, Mask R-CNN, or Detectron2 are the top choices. In laboratory settings, where fine details make a difference, Faster R-CNN has been utilised to analyse fish skin for bacterial or parasitic infections. Another noteworthy case is the use of Mask R-CNN in fish hatcheries, segmenting microscopic images to classify viable and non-viable eggs, significantly improving incubation success rates.
The choice also depends on the technical resources available. For setups with limited hardware, models like YOLO or MobileSet SSD are ideal due to their efficiency and low resource consumption. Conversely, with access to advanced GPUs, models like Dectectron2 or Faster R-CNN enable complex tasks, such as classifying multiple species in dense fish populations, helping to prevent feed competition.
Selecting the right model ensures not only efficient and sustainable operations but also the ability to address specific challenges with advanced technological solutions.