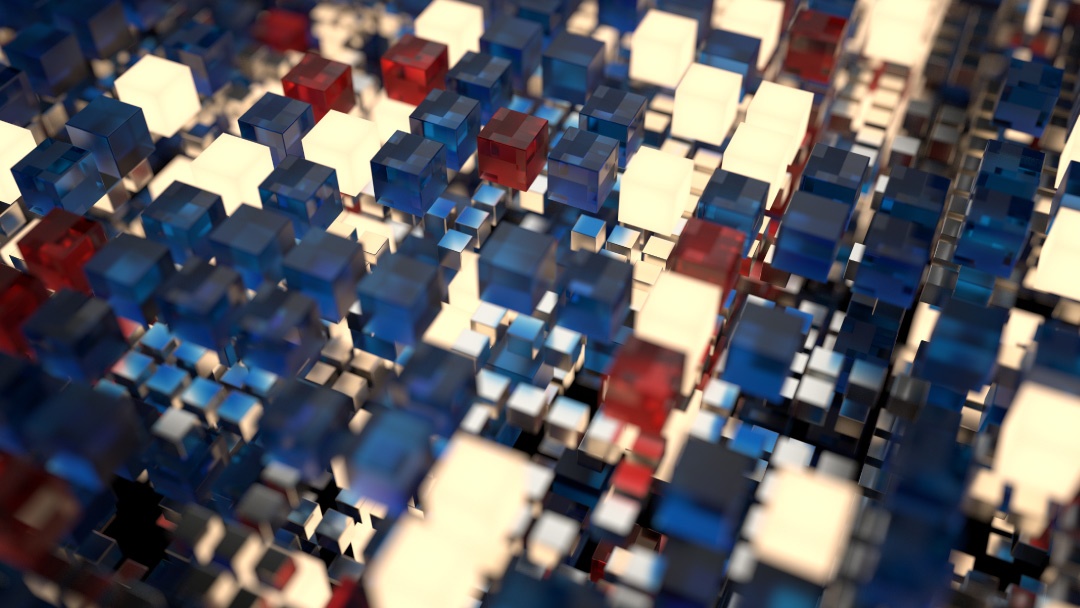
The application of neural networks, a type of artificial intelligence tool, in aquaculture genetics is still in its early stages of exploration and development. Although this tool offers greater accuracy compared to traditional methods like rrBLUP, particularly in simulation studies and certain real-world datasets, its widespread adoption in the aquaculture remains limited.
This is primarly due to challenges such as the requirement for large volumes of genetic and phenotypic data to effectively train these models.
Moreover, the costs associated with the necessary computational infrastructure and hiring specialised personnel are substantial, which has slowed its broader implementation. However, as the cost of genomic sequencing continues to decline and cloud-based services for model training become more accessible, the technology is expected to be more widely available in the coming years.
Experts highlight that while neural networks are unlikely to completely replace traditional methods in the short term, their ability to handle complex and non-linear data makes them a valuable addition to the modern aquaculture toolkit.
A recent study in aquaculture genetics demonstrates how neural networks can be used to predict resistance to koi carp herpesvirus (KHV).
The research, conducted by Christos Palaiokostas at the Swedish University of Agricultural Sciences, employed several neural networks architectures, including multilayer perceptrons convolutional neural networks, and locally connected convolutional networks.
The findings showed that, in simulated datasets, multiplayer models slightly outperformed traditional methods, albeit with a higher bias in predictions. However, when applied to a real-world dataset of carp infected with koi carp herpesvirus, traditional methods like GBLUP outperformed neural networks in accuracy by a margin of 2%.
In conclusion, while this technology is expensive during its initial phases, the investment could be justified by substantial long-term improvements in efficiency and outcomes, particularly for large-scale aquaculture operations.