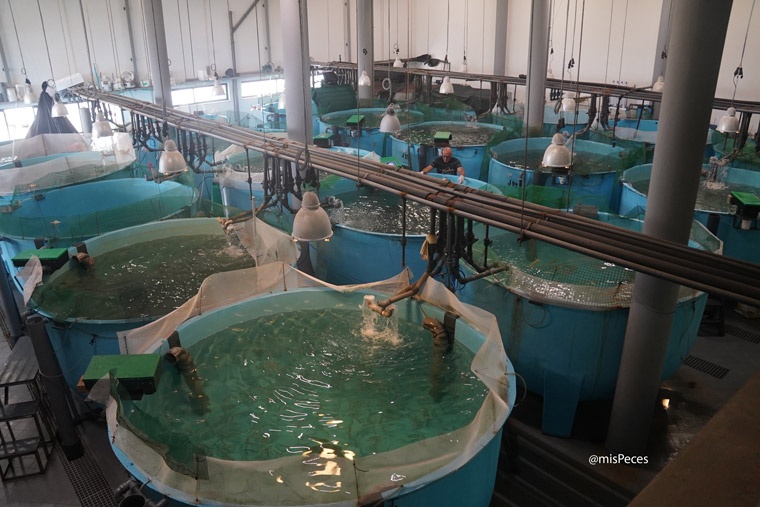
A team of researchers from the University of Porto and the Portuguese Institute for the Sea and Atmosphere (IPMA) has developed a pioneering methodology that employs machine learning algorithms to classify malnutrition in fish based on histological biomarkers of the intestine. This breakthrough, recently published at the Journal of Marine Science and Engineering, promises to transform traditional methods of nutritional evaluation in the aquaculture industry.
The study focused on analyzing intestinal sections of the meagre (Argyrosomus regius), employing both semi-quantitative and quantitative characteristics obtained through histological analysis. The algorithms used, such as logistic regression, support vector machines, and model stacking, have enabled researchers to more accurately identify signs of malnutrition. These include the number, density, and are of intestinal vili and goblet cell counts, key indicators of the fish’s nutritional state.
As the authors of this study point out, assessing intestinal health in fish farming has been complex and subject to considerable variability among studies. This new approach not only standardises this process but also makes it more accessible for operators without specialised training in histology.
This development has the potential to significantly change how fish health is monitored and managed in aquaculture facilities, offering a quicker and more cost-effective method for detecting nutritional problems before they severely impact fish populations. Aquaculture is a vital industry for global food security, and enhancements in monitoring technology like this are crucial for its sustainability and future expansion.
A Standardised Methodology for Future Developments
The researchers used juvenile meagre weighing approximately 5 grams, which were raised the Olhão Pilot Fish Farming Station (EPPO) of the IPMA.
Three different diets were formulated to evaluate various nutritional states: a control diet with normal nutrient levels and two experimental diets with decreasing nutrient levels to induce malnutrition.
After a controlled feeding period, fish intestine samples were collected, divided into anterior, intermediate, and distal section. The samples were fixed and processed using standard histology techniques to create slides that were stained and examined under a microscope.
Quantitative analyses were carried out that included measurements such as the height and area of the vili; while semi-quantitative analyses assessed general morphological characteristics and the presence of inflammatory cells.
Several machine learning algorithms were used, including logistic regression, support vector machines, and ensemble methods such as stacked assembly.
The models were trained using the training set to learn how to classify the nutritional state based on histological characteristics.
The models were evaluated on their ability to accurately predict malnutrition, and statistical analysis was performed to validate the results.
This integrated approach not only demonstrates improved accuracy in detecting malnutrition in fish but also establishes a standardized protocol that can be replicated and applied in various aquaculture environments to monitor and enhance the health and wellbeing of fish.