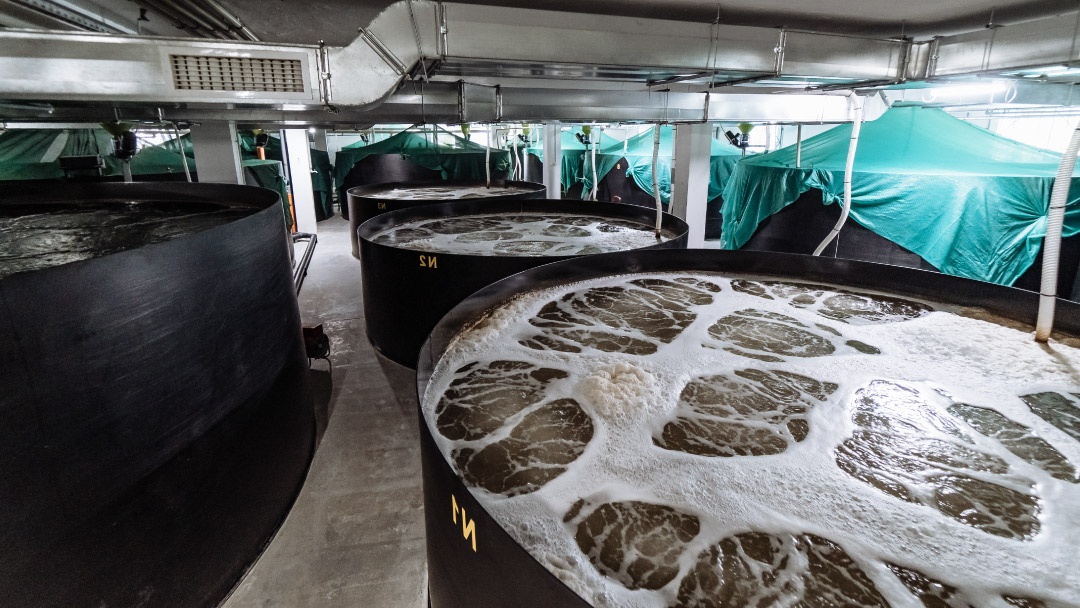
A team of researchers from Taiwan and Norway has developed ShrimNet, a state-of-the-art artificial intelligence tool designed to detect and count shrimp larvae with remarkable accuracy in dynamic aquaculture environments.
The system is built on a lightweight architecture optimized for edge devices, making it an adaptable and cost-effective solution for shrimp farms.
Led by Yo-Ping Huang, the authors explain that “ShrimpNet is based on the YOLOv8n framework and has been specially tailored to detect extremely small objects, such as shrimp larvae, which typically range from 8 to 15 mm in length.”
Traditional counting methods-manual visual inspections-are time-consuming, error-prone, and may even cause harm to the organisms. What’s more, underestimating or overestimating larval counts can significantly impact feeding strategies, effecting water quality and survival rates.
To address this challenge, ShrimpNet introduces several innovations, including the DWASNet backbone, which uses depthwise separable convolutions, channel-wise attention mechanisms, and channel mixing modules. The model’s neck structure has also been enhanced with the DPCCSP module, allowing for efficient fusion of multi-scale features.
Operational tests were carried out in two settings: containers with still water and flumes with flowing water. In both scenarios, the counting algorithm accurately identified larvae, even under difficult conditions such as overlap, rapid movement, or shifting light. “The algorithm uses zonal detection, temporal smoothing, and a fail-safe mechanism to minimise error caused by reflections or debris,” the researchers note.
In testing, ShrimpNet achieved an impressive accuracy of 97.02% and maintained an error rate below 4%, outperforming models like YOLOv8n and YOLOv9n. With only 2.7 million parameters and 12.9 GFLOPs, its low computational demand enables deployment on budget-friendly systems.
In terms of detection depth, the model remains highly reliable-above 90% accuracy up to 30 cm-though performance declines with greater depth due to turbidity and occlusion.
ShrimpNet has already been successfully tested in real-world environments, proving its versatility not only in aquaculture, but also in detecting small, densely packed objects across other sectors. The team is now working on future upgrades to extend its capabilities to deeper waters and multi-species detection.